3CPG and Comp Bio Seminar: Dr. Adam Siepel
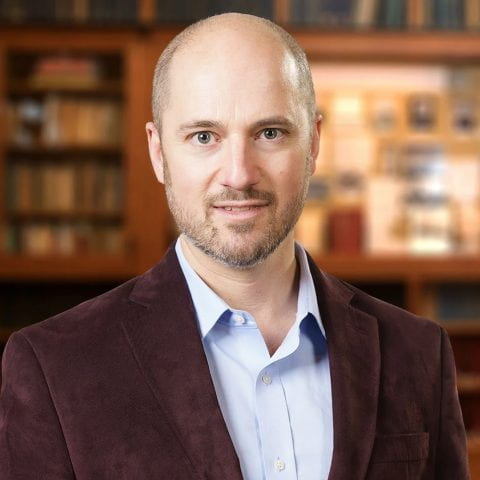
Dr. Adam Siepel, Professor and Chair, Simons Center for Quantitative Biology, Cold Spring Harbor Laboratory
“Probabilistic and machine-learning methods for problems in population genomics and transcriptional regulation”
I will describe my research group’s recent progress in developing computational methods to address two mostly unrelated problems in genomics: inference of selective sweeps from population genomic data and characterization of the dynamics of transcription from nascent RNA sequencing data. In the first part of the talk, I will describe our methods for inferring ancestral recombination graphs (ARGs) from sequence data, and then show how features from inferred ARGs can be used in a neural-network setting to improve not only the detection of selective sweeps but also estimation of selection coefficients and allele frequency trajectories. I will then present a new approach for mitigating the problem of “simulation misspecification” that arises when training neural networks of this kind, by framing it as a problem of “domain adaptation” and using a gradient reversal layer to improve generalization to real data. In the second part of the talk, I will introduce a unified probabilistic model for the dynamics of transcription initiation, promoter-proximal pause escape, and elongation, and the generation of nascent RNA sequencing read counts under steady-state conditions. I will show using simulated data that the approach yields accurate estimation of key rate parameters and correctly identifies epigenomic and DNA-sequence covariates of local elongation rates. Then I will summarize analyses of several publicly available PRO-seq data sets, showing that pause-escape is often strongly rate-limiting, that steric hindrance in the promoter-proximal region can dramatically reduce initiation rates, and that reductions in local elongation rate are associated with cytosine nucleotides, DNA methylation, splice sites, RNA stem-loops, CTCF binding sites, and several histone marks. Finally, I will introduce a convolutional neural network that improves our predictions of local elongation rates. Altogether, the talk will summarize several years of methods development in two important areas of genomics, and insights from applying these new methods to real genomic data.